How generative AI can enhance disclosure review processes
21 May 2024Head of lawtech and chief knowledge & innovation officer, Christopher Tart-Roberts, senior knowledge lawyer, Madeleine Brown, and lawtech associate Charlie Goodman have written the below article for Law 360. This was first published on 21 May 2024.
As part of the UK government's proinnovation approach to the regulation of artificial intelligence, the Legal Services Board was recently asked to outline its strategic approach to the technology.
In its response on April 29, the umbrella regulator for the legal industry recognised the increasingly important role of AI, and the need to unlock its benefits for both consumers and providers.
This echoed the sentiment of the Solicitors Regulation Authority's latest risk outlook report on AI published in November 2023, which adopted a constructive approach to innovation, acknowledging that consumers "increasingly expect firms to use technological tools that improve services." This increasingly optimistic attitude is not confined to the regulators — it is also noticeable among solicitors and barristers.
The 18 months since the launch of OpenAI's ChatGPT has afforded society the space to become more comfortable with generative AI, both in terms of its limitations and how to overcome issues such as hallucinations. This is demonstrated by a shift in responses to two major surveys.
In March 2023, Thomson Reuters Corp. found that only 51% of lawyers surveyed in the UK, US and Canada who had heard of generative AI thought that it should be used for legal work. In January, LexisNexis Legal & Professional found that around of 60% of solicitors in the UK are already using the technology or have plans to do so.
The elephant in the room is the mismatch between the almost boundless sense of opportunity and the lack of implementation to date. When Macfarlanes attended the Future Lawyer UK conference last month to hear a group of innovation leads from major city law firms discuss generative AI, it was clear that the sense of excitement that pervaded the conversation was matched by a repeated acknowledgement that the key task now is actually to put tools into production.
Now that every major law firm has provided its own take on generative AI, the critical challenge for the legal sector centers around precisely how and where to deploy it in legal work processes.
To use the terminology of Gartner's hype cycle — a popular methodology for understand the adoption of technology as it matures — we appear to have long passed the peak of inflated expectations, made our way through the trough of disillusionment, and are beginning to climb the slope of enlightenment toward the anticipated plateau of productivity, where use-cases and implementation are well understood.
In the spirit of uncovering immediate, actionable use-cases, this article will focus on the disclosure process to tease out the opportunities for using generative AI in a manner that assists lawyers, improving efficiency and thus benefiting clients. As one of the most document-intensive legal exercises, the disclosure process presents itself as a prime use-case for tools that sift through vast volumes of data, uncovering patterns and allowing users to query that information.
This article will provide a brief history of technology-assisted review, or TAR, in disclosure exercises, consider the difference between existing machine-learning models and generative AI, and explore the implications for future disclosure exercises.
Ultimately, the article will argue that generative AI will broaden rather than deepen the use of AI in disclosure. Traditional TAR has already provided significant efficiencies in disclosure, freeing up fee earner time. Generative AI is poised to help with the more strategic tasks.
Defining Technology-Assisted Review
TAR, or predictive coding, is a process whereby human expertise is blended with AI to review large volumes of documents, typically in far less time than a traditional army of paralegals.
Datasets for disclosure exercises in typical commercial litigation matters are now so voluminous that a standard "eyes-on" linear review of all documents would be impossible, or at least prohibitively expensive and time-consuming.
All forms of TAR start with a manual review of a sample set of the documents by a party's legal team, usually determining each document's relevance to the issues at play. Next, the machine-learning algorithm detects patterns in this labeled sample, which are then used to categorise the remaining documents, predicting those that are likely to be relevant and those likely not to be.
Today, the advanced TAR models work on a continuous basis, regularly rescoring documents based on ongoing human review.
The courts have long acknowledged that TAR not only has the potential to reduce disclosure costs and reduce time to trial but also has the ability to carry out the process with a higher degree of accuracy than a keyword search and human review approach only. Notably, the Business and Property Courts' rules now require parties to discuss and seek to agree on the use of TAR.
While a manual review may appear the safest, belt-and-braces approach, poring over millions of documents requires huge teams of reviewers — all with varying interpretations of the issues in dispute and the context of the case — leading to considerable inconsistencies in review decisions. In addition, human reviewers naturally tire throughout the exercise, again affecting the quality of the review output.
With TAR, the expertise of a senior lawyer well-acquainted with the case is effectively harnessed and applied across the documents in a way that would not be possible manually.
The Differences Between Generative AI and Existing Technology-Assisted Review Models
The existing machine-learning models that underpin TAR platforms are largely distinct from those used by generative AI.
TAR relies heavily on supervised learning, whereby the model is provided with a prelabeled dataset, in which the inputs are the documents and the labels are "relevant" or "not relevant." Depending on the provider, various types of neural networks, regression and pattern-recognition models may be applied. This form of AI is particularly well-suited to dealing with processes where highly complex inputs are mapped to binary outcomes.
By definition, generative AI models differ in that they create new content, focusing on replicating the patterns that they spot in phraseology, grammar and syntax, predicting the next word or punctuation in a sentence based on preceding context. This feature makes generative AI uniquely capable at tasks such as summarisation, rephrasing and drafting.
As will be outlined below, this makes it an additional tool in the disclosure process, rather than a new version of TAR.
Generative AI and the Future of Disclosure: Synthesis, Not Classification Determining Relevance
A common perception is that generative AI models pick up on nuances in the written word to a far greater extent than traditional models, such as those used in TAR. On that basis, there would be reason to believe that they would excel in document categorization, pulling ahead particularly where the document sets are smaller.
However, in reality, existing TAR models increasingly use sophisticated natural-language processing and continue to be more effective and less resource-intensive than generative AI at bulk document categorization. In testing, ChatGPT has demonstrated a particularly strong bias toward deeming documents irrelevant.
Synthesizing and Interrogating Documents
However, to dismiss the application of generative AI to disclosure due to its apparent weaknesses in relation to bulk categorization would overlook the multitude of other key tasks that feed into the disclosure exercise. There are smart ways to use generative AI's distinct ability to produce new content, beyond the document review process.
Lists of Issues for Disclosure
Under the Civil Procedure Rules in England and Wales, parties to cases in the Business and Property Courts are responsible for preparing a disclosure review document to assist the court in defining the scope of disclosure in the specific case.
The first of the three sections requires parties to formulate a list of issues that will ultimately form the basis for determining the relevance of a document. Generative AI is uniquely suited to this task.
These models can digest statements of case to produce a list of the main issues at play in a prescribed format. In the context of completing the disclosure review document, legal teams can now leverage generative AI to help condense claims, defenses and counterclaims down to the core issues at play without simply rehashing the statements of case.
Lists of Custodians
In addition to the issues at play, the scope of disclosure is determined by the relevant document custodians. In complex cases with dozens of issues spanning years and multiple parties, the task of ensuring adequate coverage while limiting the introduction of irrelevant documents is challenging.
Just as generative AI can assist with providing a first draft list of issues, it is now starting to be used to provide high-level insights such as timelines and relevant parties.
As with all use of AI, oversight from those familiar with the case is essential. However, generative AI can provide a strong basis from which to establish the relevant document sources.
Document Question Answering
Aside from the specific, process-driven exercises outlined above, generative AI also allows legal teams to interrogate their large documents in real time. While TAR models excel at classification, generative AI models excel at answering questions asked in natural language, querying underlying data without the need for query-specific training.
Over the last 12 months, rapidly increasing input limits, improvements in retrieval techniques, and a collapse in the costs associated with running these models have made large-scale queries both viable and markedly less expensive.
Enhancement, Not Replacement
Just as TAR did not displace fee earners in the disclosure process, generative AI models are poised to assist with the more strategic aspects of disclosure rather than take the wheel. No consideration of the application of AI to legal tasks would be complete without an examination of the risks.
An unavoidable concern regarding these tools, which operates using pattern recognition, pulling out correlations that are incomprehensibly intricate, is their lack of explainability.
Critically, this concern is addressed for the use-cases identified above for generative AI models through mechanisms to verify responses and generated content. Citations are now common among generative AI tools that interrogate specific documents, allowing legal teams to check conclusions.
Similarly, a list of identified issues or custodians generated from a statement of case provides an effective starting point for fee earners who are already familiar with the case, lightening the cognitive load in the same way that trainees provide effective structures for solicitors to adapt and hone.
Generative AI, used in a targeted manner, will extend the cost and time efficiencies that TAR already provides. The reliability and lower costs of existing TAR models mean that they will remain as the core technology in determinations of relevance. It is still best to use a sledgehammer to crack the nut, but generative AI will help your legal team pick through the pieces.
Get in touch
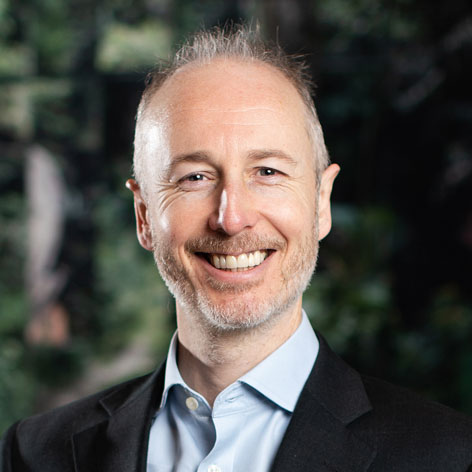